Welcome to My Research!
You may have access to the free features available through My Research. You can save searches, save documents, create alerts and more. Please log in through your library or institution to check if you have access.
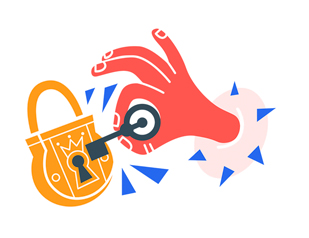
Translate this article into 20 different languages!
If you log in through your library or institution you might have access to this article in multiple languages.
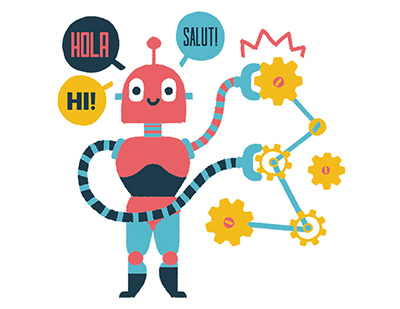
Get access to 20+ different citations styles
Styles include MLA, APA, Chicago and many more. This feature may be available for free if you log in through your library or institution.
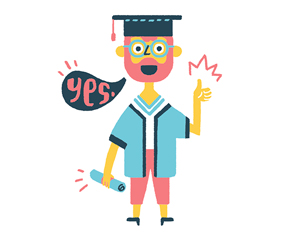
Looking for a PDF of this document?
You may have access to it for free by logging in through your library or institution.
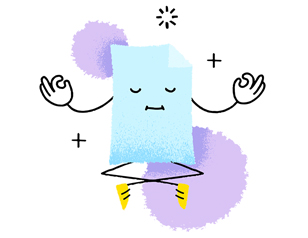
Copy link
Want to save this document?
You may have access to different export options including Google Drive and Microsoft OneDrive and citation management tools like RefWorks and EasyBib. Try logging in through your library or institution to get access to these tools.
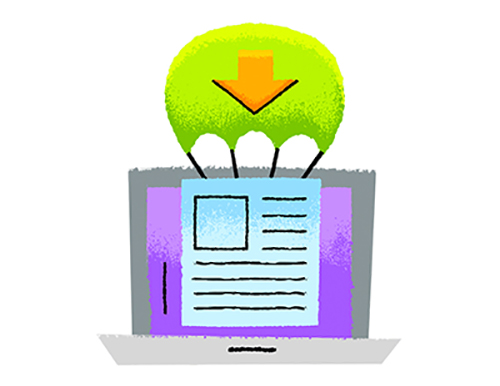

Inference-Based Personalized Recommendation Via Uncertainty-Aware Dual Actor-Critic Using Reinforcement Learning
Select results items first to use the cite, email, save, and export options
Document Section options
It appears you don't have support to open PDFs in this web browser. To view this file, Open with your PDF reader
Log in through your library to see what you might have access to.